Benefits and Challenges of Combining Data in Meta-Analysis
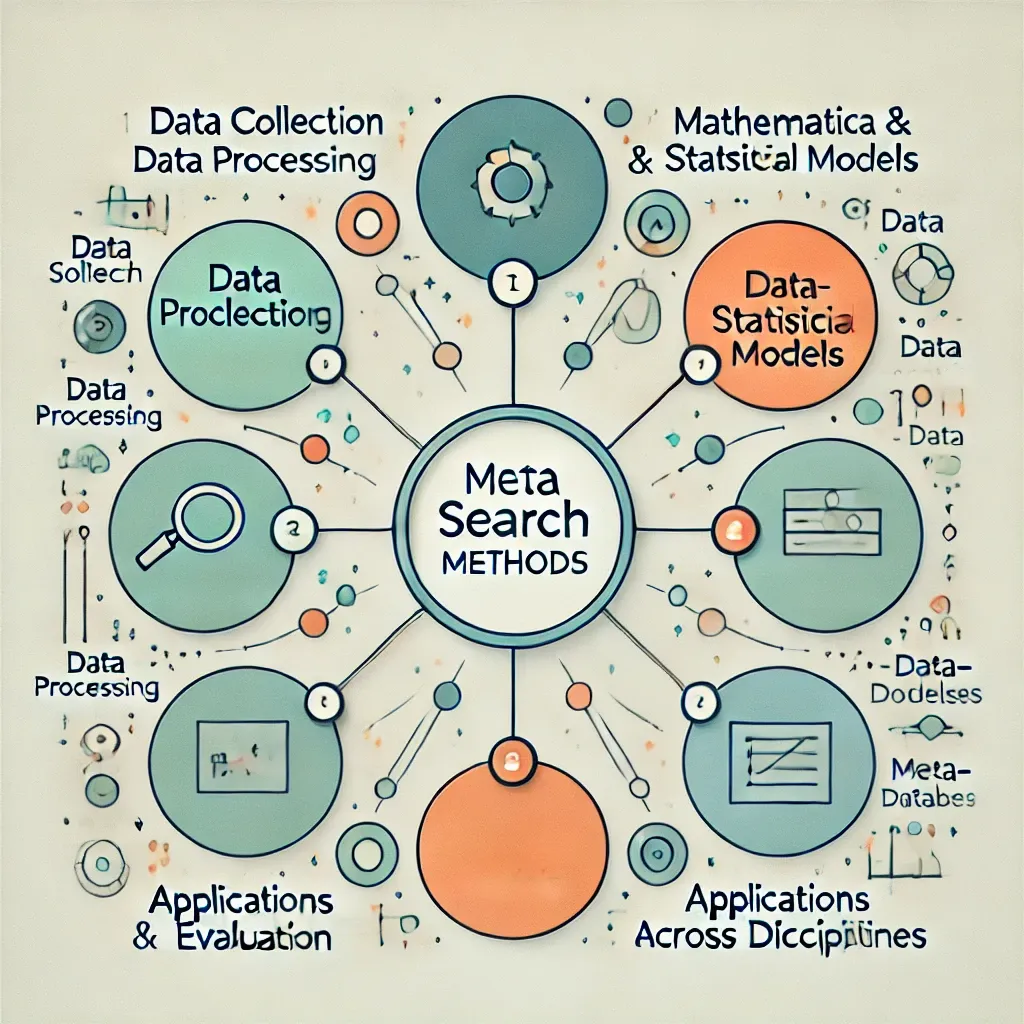
Meta-analysis offers several benefits:
- Increased precision: Combining data from multiple studies can lead to more precise estimates of effect size than individual studies, especially if those studies are small or have high variability.
- Answering new questions: Meta-analysis can answer questions that weren't addressed in individual studies by examining data across diverse populations, interventions, and outcomes. This can help researchers explore the consistency of effects in various contexts.
- Resolving controversies: Meta-analysis can help settle controversies arising from conflicting studies by formally assessing the degree of conflict and quantifying the reasons for different results.
However, there are also challenges:
- Heterogeneity: Variability between studies (heterogeneity) can be a significant challenge. If studies are too diverse in terms of participants, interventions, outcomes, or methodology, combining them may lead to misleading results.
- Bias: Meta-analysis is susceptible to various biases present in the original studies, such as publication bias (where studies with significant results are more likely to be published), reporting biases, and design flaws.
- Data extraction and preparation: Extracting and preparing data from multiple studies can be complex and time-consuming. This includes identifying relevant parameters, handling missing data, and ensuring data consistency across studies.
- Choosing appropriate effect measures: Different studies may use different outcome measures. Selecting appropriate effect measures and converting them to a common metric is crucial for meaningful comparisons.
Here are some additional details and insights from the sources:
- Types of data: Meta-analysis can combine data from various sources, including individual participant data (IPD) and aggregate data (AD).
- Statistical models: Different statistical models are used in meta-analysis, including fixed-effect models (assuming a common effect size across studies) and random-effects models (acknowledging variation in effect sizes).
- Sensitivity analyses: Sensitivity analyses are essential to assess the robustness of meta-analysis findings to various decisions and assumptions, such as the choice of statistical model or the handling of missing data.
- Qualitative meta-synthesis: Meta-analysis can also be applied to qualitative research through meta-synthesis, which involves systematically reviewing and integrating findings from multiple qualitative studies.
- Importance of context: It's crucial to interpret meta-analysis findings within the context of the included studies, considering the limitations of the individual studies and the potential for biases.
- Meta-regression: Meta-regression can be used to explore the relationship between study characteristics and effect sizes, helping to explain heterogeneity.
- Reporting quality: The quality of reporting in meta-analyses is important for transparency and reproducibility.
Overall, combining data from different studies in meta-analysis can be a powerful tool for synthesizing research findings and drawing more robust conclusions. However, it's essential to address the inherent challenges and limitations of the methodology to ensure the validity and reliability of the results.
Comments ()